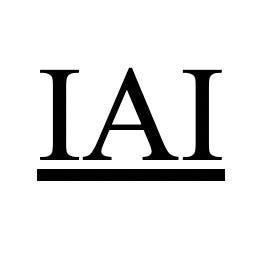
What this work shows is that if you have the right input basic material (data) with the right distribution (here, a heterogeneous one across a bunch of robots), and then you train a high-capacity neural net on it, you get out something greater than the sum of its parts - a model with surprisingly good out-of-distribution generalization as a consequence of some critical reaction that occurs due to your combo of data + architecture + complexity.
Sometimes I think that developing AI is more like a chemical process rather than a machining one.
Josh Beckman